Project P4
Deep learning and pathomics augmented nephropathology
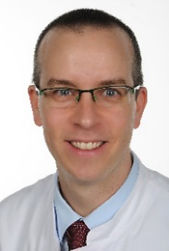
Univ.-Prof. Dr. med. Peter Boor, PhD, MD (Spokesperson)
Institute of Pathology
Department of Nephrology
University hospital RWTH Aachen
E-Mail: pboor@ukaachen.de

Univ.-Prof. Prof. h.c. Dr.-Ing. Dorit Merhof, PhD
Institute of Imaging & Computer Vision
RWTH Aachen University
Email: dorit.merhof@lfb.rwth-aachen.de

Nephropathology is essential for the diagnosis of renal diseases and a major read-out of experimental pre-clinical kidney studies. The advancement in digital pathology , i.e. digitalization of histological slides, and the application of artificial intelligence, especially deep learning, has opened new research perspectives that have the potential to transform diagnostic pathology into quantitative “computational” pathology. We leverage our state-of-the-art high-throughput digital computational pathology infrastructure and interdisciplinary expertise to develop and apply deep learning for clinical and experimental nephropathology. We will address various challenges and limitations, including the unavailability of manually annotated data and staining variations, by developing semi- or un-supervised and stain-independent approaches. Additionally, we will focus on large-scale extraction of quantitative image features that might detect previously unrecognized morphological attributes, i.e., “pathomics”. Besides, we will provide our nephropathology and image analyses expertise for the whole consortium.
In conclusion, we aim to develop and apply deep learning and pathomics approaches to facilitate innovative quantitative pathology diagnostics towards a more precise and personalized nephropathology.
Boor P. Artificial intelligence in nephropathology. Nat Rev Nephrol. 2020, 16:4-6.
Gadermayr M, Gupta L, Appel V, Boor P, Klinkhammer BM, Merhof D. Generative Adversarial Networks for Facilitating Stain-Independent Supervised & Unsupervised Segmentation: A Study on Kidney Histology. IEEE Trans Med Imaging 2019, 38: 2293-2302.
Bouteldja N, Klinkhammer BM, Bülow RD, Droste P, Otten SW, von Stillfried S, Moellmann J, Sheehan SM, Korstanje R, Menzel S, Bankhead P, Mietsch M, Drummer C, Lehrke M, Kramann R, Floege J, Boor P* & Merhof D*. Deep-Learning based multi-disease, multi-species, multi-class segmentation and quantification of kidney histology. J Am Soc Nephrol. 2020, accepted PLEASE UPDATE. [*joined senior authors]
Kers J*, Bülow RD*, Klinkhammer BM, Breimer GE, Fontana F, Abiola AA, Hofstraat R, Corthals GL, Sengers HP, Djudjaj S, von Stillfried S, Hölscher DL, Pieters TT, van Zuilen AD, Bemelman FJ, Nurmohamed AS, Naesens M, Roelofs JJTH, Florquin S, Floege J, Nguyen TQ, Kather JN, Boor P. Deep Learning-based classification of kidney transplant pathology: a retrospective, multicente, proof-of-concept study: Lancet Digit Health 2022 Jan;4(1):e18-e26. doi: 10.1016/S2589-7500(21)00211-9. Epub 2021 Nov 15.
Selected Publications